Making the Business Case for the CECL Approach – Part III [White Paper]
Table of Contents
Skip to table of contentsKey Takeaway
We provide ALM and CECL solutions that help our clients measure, monitor, and mitigate balance sheet risk on an integrated basis. We consider credit, interest rate, and liquidity risk holistically. We charge a fee for our advice and do not rely on commissions, so we can remain objective. We simply want what is best for our client.
How Can We Help You?
Founded in 2003, Wilary Winn LLC and its sister company, Wilary Winn Risk Management LLC, provide independent, objective, fee-based advice to over 600 financial institutions located across the country. We provide services for CECL, ALM, Mergers & Acquisitions, Valuation of Loan Servicing and more.
Released June 2017
Introduction
This white paper is the final in a three-part series. We believe the implementation of CECL presents financial institutions with the opportunity to better integrate credit risk analysis across the organization. While it is imperative to understand expected life-of-loan credit losses in conjunction with the accounting requirements of CECL, this paper makes the business case that forecasted life-of-loan credit losses can be used to better manage an institution.
Our first paper in the series demonstrated how life-of-loan credit loss analytics can be used to perform capital stress testing, as well as to evaluate the risks and opportunities of potential business strategies. The second showed how life-of-loan credit loss estimates can be used to develop quantitative concentration limits. This paper details how life-of-loan credit loss estimates can be used to properly price loans for risk under changing macroeconomic conditions.
We believe the best managed institutions measure, monitor, and manage credit, interest rate and liquidity risks on an integrated basis as part of their ongoing ALM process.
We believe incorporating lifetime credit loss estimates under multiple economic environments will ultimately lead senior management to better comprehend the risks and opportunities of its financial institution’s balance sheet and operating model. It enables an organization to chart a path to maximize it potential profits while, at the same time, ensuring credit risk exposure and its potential detrimental effect on an organization’s capital is actively measured, monitored and controlled. This concluding white paper focuses on utilizing lifetime credit loss estimates to improve loan pricing margins based on expected real return analyses. We demonstrate how financial institutions can appreciably enhance their loan pricing margins by prospectively considering how credit risk changes as economic conditions change and quantifying the potential effects on its real margins. Expected real return analyses provide immediate feedback on current pricing methodologies, highlight areas where improvements are needed and ultimately lead to a better understanding of the risk/return tradeoff.
Expected Real Returns
Loan interest rates can be expressed in two ways: nominal rates or real rates. While real rates of return are often used in the context of inflation, in this case “real rates” are based on the income a financial institution earns on loans after adjusting for expected credit losses. The expected real return is determined by calculating the internal rate of return of the cash flows expected to be received over the life of the loan. In addition to loan attributes such as term and interest rate, the expected loan cash flows are dependent on model input assumption estimates for:
- Voluntary prepayment – conditional repayment rate (“CRR”)
- Involuntary prepayment – conditional default rate (“CDR”)
- Loss severity if a default occurs
To best apply these assumptions in a real return analysis, Wilary Winn first stratifies the portfolio by loan attributes. For example, we separate first lien residential real estate loans from junior lien loans. We further divide the first liens into fixed and variable, and junior liens between closed and open-ended. For auto loans, we separate new from used, and further segment by direct and indirect. We do this because our modeling experience has shown that these loan types perform differently from a credit loss perspective even with similar credit indicators. If the source of the loan is indirect, we also subtract any dealer fees associated with the loan in performing real return analyses.
We then segment the loan categories by predictive credit indicators to best estimate involuntary prepayment and loss severity. For residential real estate loans, we use FICO and combined loan to value. For other consumer loans, we rely primarily on FICO. For commercial loans we typically apply the credit assumptions by risk rating, debt service coverage and NAICS code.
In addition to analyzing lifetime credit losses in a base case economic environment, we believe real return analyses should also consider the sizeable additional lifetime credit losses that could occur if adverse economic conditions were to arise, such as an extended period of high unemployment. Conditional default rates increase exponentially in adverse economic environments, particularly for the low credit groups. Even if the current pricing matrix makes financial sense in the current economic environment, we believe financial institutions should include realistic worst-case scenarios in performing real return analyses and examine returns in a stressed environment. Often, Wilary Winn’s real return analyses show negative returns for low credit cohorts when analyzed under stressed economic conditions.
At Wilary Winn, we calculate expected real returns by subtracting the weighted average estimated lifetime credit loss percentage at the loan cohort level from the weighted average interest rate. The resulting analysis highlights the estimated profit margin that each pricing tier is contributing and confirms whether or not each loan cohort pricing group is appropriately priced for any given set of economic conditions. We believe that the advantages of incorporating real return analyses into senior management strategy discussions are:
- Improved understanding of the return outcomes by loan cohort
- Early recognition of potential errors in the pricing model
- Increased understanding of credit loss exposure in multiple economic environments which helps determine the amount of capital an institution is willing to put at risk
- Identification of the “sweet spot” in the pricing matrix which leads to superior returns and potentially leads to market differentiation
- Enhanced communication between the loan origination and finance departments
Real Return Analysis
The starting point of a real return analysis is the financial institution’s current pricing grid for a given loan product. By way of example, the following table shows an organization’s used vehicle indirect pricing matrix.
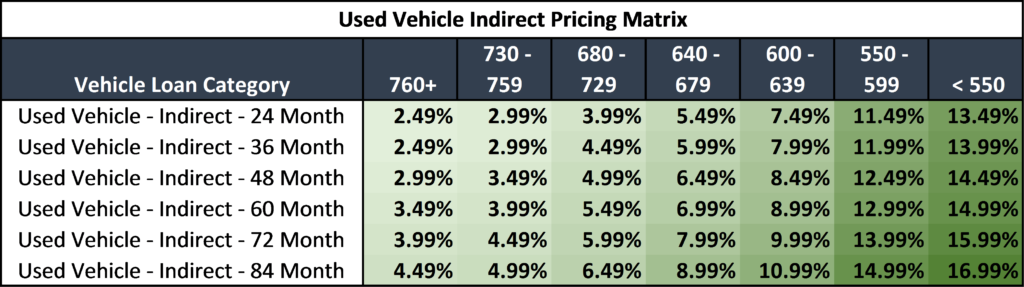
The table shows that loans made to borrowers with lower FICO scores are made at higher interest rates designed to cover the increased credit risk associated with the borrower with less favorable credit quality. Additionally, we note that institutions generally charge more for loans with longer maturities both to cover the risk-free rate and to cover for increased credit risk. The longer the loan term, the higher the probability is of loan amortization not keeping up with vehicle depreciation, which in turn increases the credit risk exposure.
The next step in the real return analysis is to calculate the internal rate of return of the expected cash flows to be received over the life of each loan pricing cohort. We begin with the contractual cash flows, apply our CRR assumption to account for voluntary prepayments, and apply our CDR and loss severity assumptions to account for credit losses resulting in cash flows expected to be collected. We then compare the expected cash flows to the beginning loan amount to calculate the internal rate of return. The reductions to the nominal rate of interest thus include estimated future credit losses, lost interest on delinquent loans, and indirect dealer fees associated with the loans. As presented in the following table, the estimated real returns are lower than the nominal interest rates in the loan pricing matrix due mainly to application of the credit loss assumptions. We note that our calculation results shown are based on favorable economic conditions similar to those of today.
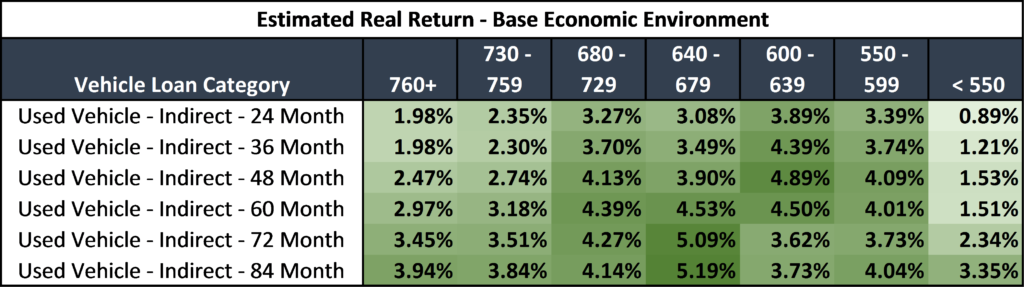
Overall, the financial institution’s risk-based pricing matrix adequately covers estimated credit losses across all the FICO and loan term cohorts. The 760+ FICO, low term cohorts reflect an estimated real return that is lower in comparison to most other credit tiers. This result is most likely due to increased competition for the highest FICO, lowest term borrowers. It is worth noting that the probability of experiencing a credit loss and overall credit loss volatility is extremely low in these categories thus potentially justifying the lower overall returns. The previous table also shows that the estimated real returns tend to increase as FICO scores decrease until credit scores are under 640 with loan terms of 60 months or greater. This illustrates the increased risk associated with lending over a long term to lower credit quality borrowers. The real return chart clearly shows that the nominal interest rates charged are adequate given the expected credit loss performance under the current, favorable economic environment as all values are positive.
We mentioned that one of the benefits of creating a real return analysis was to determine the “sweet spot” for the financial institution. Wilary Winn believes that the “sweet spot” is the place where the highest overall return levels are found. This determination is based on examination of each respective FICO, term cohort and its related expected real returns percentage. As shown previously in the base case economic environment estimated real return table, the highest expected real returns the financial institution experiences are found at various term lengths on the 680-729, 640-679, and 600-639 FICO cohorts.
The final step in the real return analysis process is to examine the estimated real returns in a stressed economic environment scenario. The following table provides estimated real returns under a stressed economic environment. In this case, we have assumed that our maximum economic stress is a sustained unemployment rate of 10.2%, the highest unemployment rate experienced during the most recent business cycle. Our conditional default rate for this stress analysis was adjusted based on the historic statistical relationship between the unemployment rate and used vehicle loan defaults.
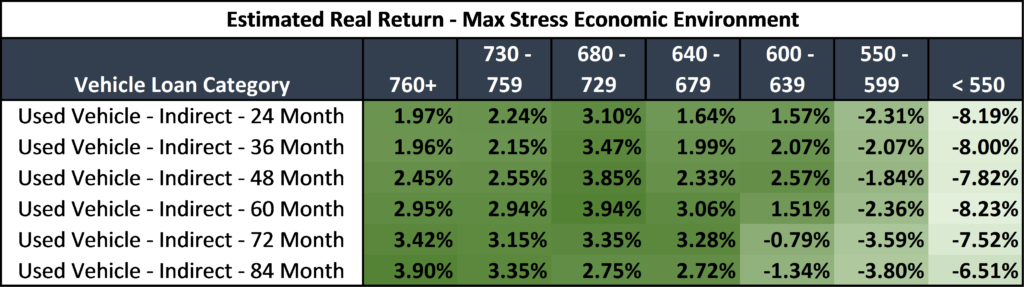
Not surprisingly, under the stressed economic environment, overall estimated real returns decreased in every loan pricing cohort. The previous table shows an appreciable decrease in estimated real returns with FICO scores under 640. We note that the financial institution’s nominal interest rates are inadequate to cover credit losses starting at the 600-639 FICO cohort with continued and increased degradation at the 550-599 and <550 FICO cohorts. WW Risk Management’s model results show a marked increase in the vulnerability of the expected real returns as FICO scores decrease. This depiction is logically consistent with an adverse economic environment because the unfavorable economic conditions tend to have an amplified and non-linear impact on borrowers with lower credit scores.
The result of the stressed economic analysis is a revised “sweet spot” on the pricing grid. Wilary Winn believes that the “sweet spot” concept is one of the most important benefits of the real return analysis. With the knowledge of what type of loan cohort is producing the largest returns after credit losses, the institution can implement different techniques to attract more of this particular market segment and increase overall earnings and capital. The following table depicts at a high level how a financial institution models increased production in certain cohorts to ultimately analyze profitability, risk and balance sheet impact.

Wilary Winn believes a financial institution can improve its overall returns by iteratively performing real return analyses. By testing returns in different economic environments, a financial institution can increase its knowledge on credit risk for each pricing cohort and potentially limit its exposure in lower credit categories as these categories are exponentially more sensitive to challenging economic conditions. Alternatively, it can increase the interest rate it charges to a level commensurate with the risk. In addition, if it faces adverse economic conditions in the future, the institution will be better prepared by knowing which strategies it needs to implement to offset the risk caused by the new economic conditions – including the changes it can make to its pricing matrix. For example, by increasing interest rates for the FICO and term cohorts that were affected the most in order to compensate for the losses it could incur in the new economic environment.
Conclusion
Financial institutions that are not SEC filers must become compliant with the required CECL provisions by 2021. Early adoption of CECL is permitted in 2019. Wilary Winn believes that the implementation of CECL presents an opportunity for financial institutions to better integrate credit risk across the organization and that there are numerous business advantages offered by the creation of additional analyses built from the lifetime credit loss calculation required by CECL. Part III of our three-part series on making the business case for CECL demonstrated how the CECL approach can be used to optimize risk-based pricing strategies through real return analysis. By examining the real returns each loan pricing cohort is producing, the financial institution can notice immediately if any cohorts are in need of updating, especially those producing negative returns. Early recognition of pricing model errors and improvements in the pricing matrix can lead to an increase in overall returns and capital.
Wilary Winn believes that real return analysis process has the potential to improve communication between the loan origination and finance departments. The real return analysis results gives the loan origination and finance departments immediate feedback on the potential profitability impact that each pricing cohort has on the overall finances of the financial institution. Examining potential losses under multiple economic environments helps key decision makers optimize the risk/return trade-off for the organization. We believe that collaborating on real return analyses can bring both groups closer together in achieving the institution’s overall financial goals.
Attend any recent or upcoming financial institution conference and you will find considerable discussion and debate about the new accounting guidance related to the Current Expected Credit Loss Model (CECL) standard. Because the standard requires a prospective estimate of lifetime credit losses, we believe the best managed institutions will incorporate the credit risk information into additional analyses to help set the strategic direction of the financial institution. Even if the CECL standard were to be withdrawn, Wilary Winn would continue to incorporate credit loss modeling as part of ALM to help our clients achieve best practices with loss exposure analysis, capital stress testing, business strategy evaluation, concentration risk management and risk based pricing and real return analysis.